Thoughts on AI Drug Discovery
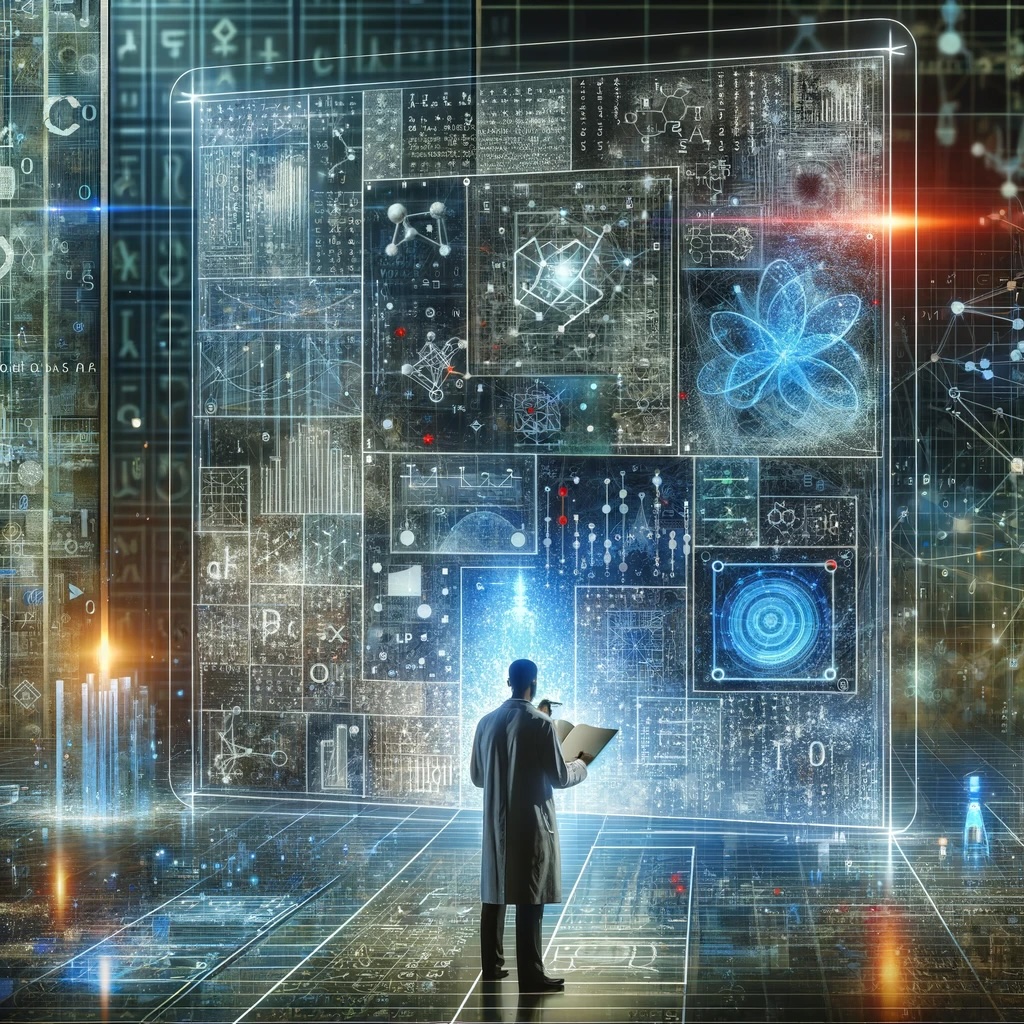
The Event
On April 25, 2024, an event titled "Bridging Human Creativity with Digital Innovation for Drug Discovery" took place in Korea. Sponsored by several companies collaborating on Daewoong Pharmaceutical's research portal project, the day featured morning sessions from Daewoong Pharmaceutical and Merck, followed by detailed afternoon discussions from Merck, P&D Solutions, T&J Tech, and Leaders Systems covering software solutions from Synthia, Spotfire, Cresset (Torx), and Chemaxon, and GPU servers.
During Dr. Kyung Seok Oh's presentation, the day's first talk, I reflected on the journey of AI in drug discovery over the past decade. It seems that while no singular method is universally correct, each approach must be tailored to fit specific circumstances and environments.
At Daewoong Pharmaceutical, they differentiate between the commonly used Target Product Profile (TPP) in drug discovery programs, which requires experimental validation, and their Target Design Profile (TDP). The TDP allows for the pre-experimental assessment of a compound's design quality, following a systematic process: Input (Chemical Structure) → Calculation (using all available models) → TDP → Decision Making. To efficiently manage this workflow, they have developed the Daewoong Discovery Portal. However, I wish to focus on some foundational questions rather than the technical specifics. Before diving into the questions, I would like to appreciate all the speakers and sponsors for this wonderful event.
Firstly, how do we balance throughput and precision in computations?
During the Calculation phase, compounds are evaluated using various techniques and models. A key challenge arises when multiple models predict the same endpoint. If the models operate independently, a voting mechanism might work, but confirming model independence is complex.
Moreover, there's an inverse relationship between computational efficiency and accuracy. For instance, even optimizing many programs and algorithms for docking simulations to predict in vitro activity is complex. More computational resources generally lead to greater accuracy. Yet, determining the appropriate level of computational investment for evaluating an idea is crucial. This depends on whether the priority is to quickly filter out ineffective ideas or to further explore promising ones. The timing of feedback after inputting a compound structure also varies based on the urgency of results, influencing how computational resources are allocated across different components of the TDP.
Secondly, what data should be presented to users inputting structures?
Defining a TDP aims to standardize decision-making based on structured data evaluation. However, achieving consensus on what data to show is challenging. Presenting too little data may undermine confidence in decisions, while too much can complicate decision-making processes. Ultimately, data visualization needs to be strategic, aligning with how decision weights are assigned within the TDP. Practical approaches might involve prioritizing projects based on their competitive stance in drug discovery pipelines.
Thirdly, can limited experimental data really enhance model performance?
This query was inspired by Dr. Youngrak Cho from Ligachem Biosciences long time ago. While model performance generally improves with more data, many projects cease after initial tests fail to yield promising results. The need for effective predictive models is most critical at the project's inception when data are scarce. Early experimental data typically involves protein binding assays without testing ADME/Tox properties outlined in the TDP.
The iterative cycle of idea → decision → experiment → confirmation should theoretically refine our evaluation models with accumulating data. Nevertheless, decision-making often incorporates factors beyond computational results such as reagent costs, procurement times, and external information (e.g., publications and peer feedback). In addition, the reason for synthesizing a compound is not just "because I think it's good" but also "for comparison", adding complexity to the decision-making and data collecting processes.
(A sort of) Conclusion
In essence, every challenge in this field could boil down to finding the right balance: How much generalization is beneficial in practice?
Although AI Drug Discovery tools are predominantly operated by humans, envisioning a model where AI autonomously makes all decisions remains speculative. The effectiveness of AI in drug discovery must ultimately be measured by the quality of resulting drugs, which remains distinct from AI performance itself.
These complex questions may not have straightforward answers but are crucial for advancing discussions in this field.
Comments